Coffee Break with AI is brought to you by Martijn Loos and Elisio Quintino.
We believe that the value of a technology should be measured by how it improves people’s lives. Although this may be cliché, it’s nonetheless important to us. Of course, technology for technology’s sake can be amusing to study and play with, but at the end of the day, it is less meaningful if it does not bring benefits to society.
In this edition of Coffee Break with AI, we would like to discuss the contributions of artificial intelligence to the protein folding problem. Solving this problem brings benefits such as a better understanding of conditions like Alzheimer’s and Parkinson’s, as well as the ability to develop powerful drugs and cure diseases like cystic fibrosis through protein design.
In this blog, we’ll introduce protein folding. But, in order to fully appreciate the topic, it’s beneficial to understand the importance of proteins first. Afterwards, we’ll present arguably the most impactful contribution of AI to the field until now.
Why should you care about proteins?
Let’s start by saying that your life depends on proteins – on a much higher level than you might think! We learn very early at school that protein-rich food is essential for building muscles and other tissues (anyone recalling the GO-GROW-GLOW food lectures?), but this is only one of many reasons to take a closer look at these molecules.
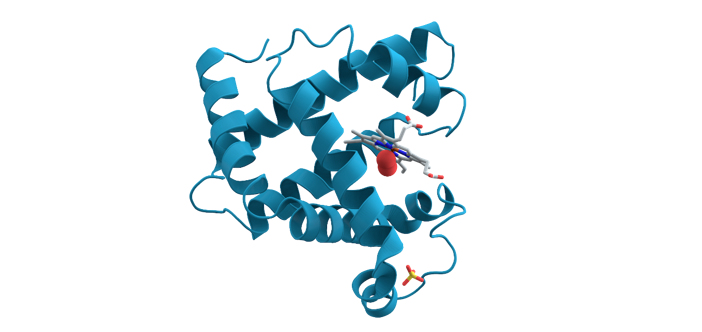
Figure 1: Myoglobin, a type of protein found in the muscle tissue of vertebrates. Source: https://en.wikipedia.org/wiki/Myoglobin
To begin with, the vast majority of enzymes are proteins. Enzymes are facilitators for chemical reactions in the body, which makes them crucial to a broad spectrum of bodily functions, such as energy production, digestion and muscle contraction. For example, eating and digesting would be practically impossible without enzymes.
Equally important, other proteins, acting as hormones, work as messengers inside the body. Growth of various body tissues is stimulated by one of these proteins, called the human growth hormone, while insulin is the hormone protein that signals the transport of glucose into cells. Issues related to the latter may cause the life-long condition diabetes.
The complete list of proteins and their importance to our body is long and beyond our scope here, but the core idea is that proteins and their understanding are crucial for life.
How proteins work and the protein folding problem
The function of a protein is tightly related to its shape. The 3-D form of each protein molecule will determine what it can or cannot do, almost in the same way that screwdrivers are good for screwing a screw, while hammers are probably best left for nails.
In its turn, the shape of a protein is strongly dictated by how its building blocks, called amino acids, are organized. A protein is a linear chain formed by a sequence of amino acids, which can be of 20 different types. Depending on the configuration of this sequence, the chain will fold together in specific ways, originating unique 3-D shapes. The core of the protein folding problem is understanding how the folding will occur based on the sequence of amino acids.
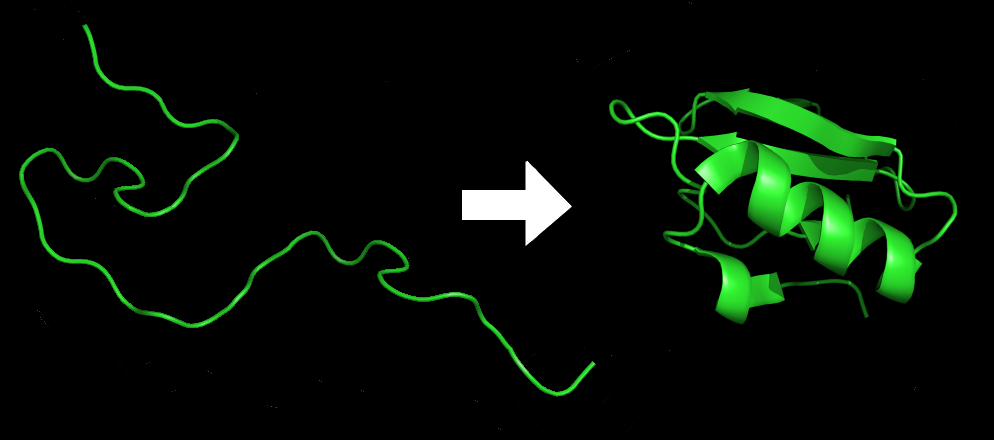
Figure 2: Protein folding process. Source: https://en.wikipedia.org/wiki/Protein_folding
Solving the protein folding problem has a series of practical and life-changing applications. We’re talking about understanding how some diseases, like Alzheimer’s, are originated, which can make it easier to develop a cure or treatment. We would also be able to better synthesize proteins with very specific properties in order to perform tasks in our body. The impact in terms of saving and improving lives is huge!
Where does AI fit in?
Researchers and scientists have been working on determining the 3D shape of proteins for the past 50 years, depending heavily on experimental techniques (such as x-ray crystallography and cryo-electron microscopy). But experimental techniques are slow and cost a lot: we are talking years of work and tens or even hundreds of thousands of dollars per protein structure.
As an alternative, computational methods have been developed to tackle the protein folding problem, depending less on trial-and-error than experimental methods and reducing the time cost magnitude from years to weeks or even days.
In this context, enter AlphaFold, an AI system developed by DeepMind (nowadays part of Google), the AI company behind the success of AlphaGo (AI system which mastered GO) and AlphaStar (AI system which learned to play StarCraft).
AlphaFold amazed the scientific community in 2018 when it showed outstanding performance in the 13th edition of CASP (Critical Assessment of Protein Structure Prediction), which aims to assess the quality of protein structure prediction algorithms.
On that occasion, it was awarded first place in the event’s rankings. The result is even more astonishing if we look closer at some numbers: AlphaFold could predict 25 out of 43 protein structures provided by CASP, while the second place could only predict three. This result puts AlphaFold in the biomedical community’s spotlight for years to come.
More recently, a newer version of AlphaFold produced structure predictions for six proteins associated with the strain of coronavirus that causes COVID-19. This could be of huge value in understanding and fighting the disease.
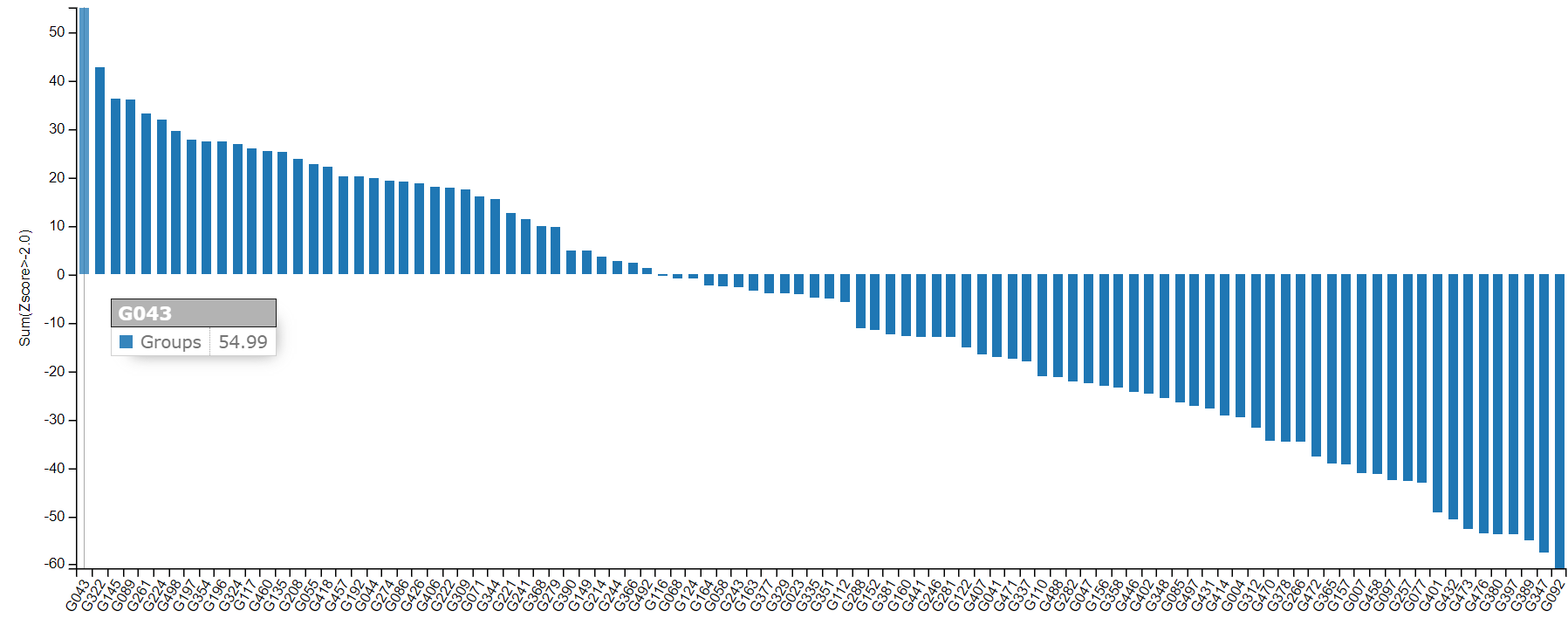
Figure 3: CASP3 results, with AlphaFold (G043) highlighted in first place. Source: http://predictioncenter.org/casp13/zscores_final.cgi?formula=assessors
Some AI concepts to take home
A full analysis of how AlphaFold works is more of a matter for a 5-course dinner than for our humble Coffee Break, and a deeper dive into the project is provided here by Andrew Senior, team leader for the project. However, we can still point to a couple of interesting concepts present in their solution which relate to AI in general.
Machine Learning in AlphaFold’s system
The first concept is the usage of machine learning in AlphaFold’s system.
Since even an approximation of all molecular interactions happening in a protein is virtually impossible to obtain, the system uses a machine learning approach to estimate the relation between sequences of amino acids and the resulting distances between them. These distances will then be processed by other parts of the system to finally obtain shapes and other information about the protein.
Neural networks have been successfully deployed by DeepMind (and the community in general) to solve increasingly hard machine learning problems. This time, the team chose a type of convolutional neural network. The term convolutional refers to convolutions, classic building blocks in modern neural nets. In the computer vision field, for example, convolutions allow the neural net to consider a dog in the left upper corner of an image as much a dog as one in the lower edge of it (a property called translation invariance).
Data Augmentation
Another technique used by DeepMind is data augmentation. Data augmentation is when more data is generated from the original datapoints with the goal of enhancing the training process of a machine learning model.
Machine learning algorithms are dependent on data for their training, and neural networks are especially data-hungry, so increasing the dataset is usually beneficial and quite often is what allows a project not to stop due to lack of data.
As an example, in object classification and object recognition problems, rotating the image of an object to generate a different image which still represents the same object (thus has the same label) is a common data augmentation technique. In the case of AlphaFold, instead of looking at the whole protein chain as a datapoint, the chains were cropped in multiple ways, resulting in many possible datapoints per protein chain. This process provided expanded datasets as sources for the training phase.
As you might already have noticed, AlphaFold is not simply an algorithm, but a system that exploits different techniques and tools to achieve a goal. In general, this is indeed the recommended approach when considering the practical use of AI – first defining a problem and then considering AI and machine learning as additional toolsets when designing the solution.
What are we looking forward to?
The 14th edition of CASP competition will be held this year. At the same time that we should hope for AlphaFold’s consistency and even improvements on their previous results, it would be great to see even more AI-powered players entering the competition, possibly inspired by DeepMind’s success. Advances in one of the most important problems in biomedicine should be a high priority for researchers in the field.
IFS Labs will be keeping a close look at AlphaFold’s way ahead, since developments in artificial intelligence algorithms, practices and systems translate in broader and more robust toolsets for us when designing solutions for IFS customers.
Learn more about the exciting work of IFS Labs here.
Coffee Break with AI is brought to you by Martijn Loos and Elisio Quintino.
Do you have questions or comments?
We’d love to hear them so please leave us a message below.
Carlos Carvalhal
Congrats. Well written and quite interesting.